In this episode of the Leader Generation podcast, Tessa Burg talks with Lilith Bat-Leah about the pivotal role of data science in marketing and various industries.
They discuss the growing significance of data science and machine learning, emphasizing the importance of data quality, domain expertise and innovation in data-centric AI applications. Lilith also shares practical advice for embracing change and the belief that AI and ML solutions don’t need to be perfect to provide value in marketing.
“When considering AI and ML solutions, they may not be perfect. But neither are humans or our existing processes, so give technology a chance.”
Expect valuable insights from Lilith’s expertise, including useful guidance for marketers looking to effectively leverage AI and ML.
Leader Generation is hosted by Tessa Burg and brought to you by Mod Op.
Highlights From This Episode:
- Importance of data science today
- Role of data scientists
- Data Analysts vs. ML Engineers
- AI impact on Marketing
- Examples of automations with ML
- Data-centric AI
- Tips for increasing AI/ML success
- Change management in AI adoption
- AI opportunities for marketers to explore
- Gender in AI and tech
Watch the Live Recording
Full Episode Transcripts
Tessa Burg: Hello, and welcome to another episode of Leader Generation, brought to you by Mod Op. I’m your host, Tessa Burg, and today I’m excited to welcome Lilith Bat-Leah. She’s the VP of Data Services here at Mod Op and leads our data science discipline. Lilith, thank you so much for joining us today.
Lilith Bat-Leah: Thank you for having me.
Tessa Burg: So first, I love your background story, so let’s start there. Tell us a little bit about yourself and your role here at Mod Op.
Lilith Bat-Leah: Sure. So, I’ll start with, with my love of statistics in school. So, I just, I really fell in love with statistics and then meanwhile, my, my career got started in the legal tech industry, and then all of a sudden machine learning for e-discovery became a big thing. And I was one of the few people with, with deep statistics expertise and the, the domain expertise in terms of, of the legal text space or, or the very specific e-discovery space that I was in. So that, that took me to my first data science role. And right in what we’re focused on is modeling subjective human judgment on, on documents that may be evidence in litigation. So, it’s classifying documents as relevant to the litigation or not relevant and right. And documents can be relevant for any number of reasons. In some cases, they could be relevant for any one of a hundred different reasons, but you were building a model that could capture all of that and predict whether a given document was relevant or not. So that’s my background. I, I transitioned out of Legal Tech joined dPrism, which was recently acquired by Mod Op to become a bit more of a generalist. So, I, I just love solving problems with ML and data science. I love improving decision-making. I’m really passionate about decision intelligence, which is is something that kind of came out of Google as, as a new discipline. And it’s all about how do you make better decisions with data? How do you think critically about the kinds of decisions that you’re automating with machine learning and, and other tech. So, so yeah. So that’s, that’s my background. I I meandered a bit there. Feel free to focus me at, at any point. Oh, no,
Tessa Burg: You were, yeah. So, let’s, I think it’s awesome that you were here at Mod Op. The other thing that is great, especially myself coming from a data background is now people have a better understanding of where machine learning and AI really adds value to marketing. But tell me a little bit more, like, why is it great to be a data scientist today?
Lilith Bat-Leah: Well, it is, it is kind of the hottest field right now, right? We’ve had this explosion. Everyone’s really excited about what’s possible and there is so much low-hanging fruit, right? It’s, it’s possible to make a big impact in the space because it is such a nascent field and, and it’s only now that things are becoming possible that people had theorized about, it’s only now that we have the compute to be able to actually put, put all those ideas into practice and, and realize all these tremendous gains.
Tessa Burg: Yes. And I think some marketers would be tempted to hire data scientists and be like, oh my gosh, AI and ML, I totally buy and believe it’s going to affect everything we do. I’m gonna hire a data scientist and put them into my analytics team. Can you help clarify for anyone who might be listening, what is the role of a data scientist versus someone who might be working also in data and an important job, but more in an analytics role?
Lilith Bat-Leah: Yeah, and I think the term data scientist is, is starting to fall out of favor because you see people landing in either the bucket of data analysts where you’re really focused on, on using data and ML to make better decisions, to monitor KPIs, to, to have this just very information synthesis focus on, on data. And then you have ML engineers where it’s really all about building products or building tools that automate a lot of decision making or, or, you know, do something just enable a workflow using machine learning. And those are two very different things, right? The, the information or data analysis and then the machine learning engineering side of things.
Tessa Burg: Yeah, and I think, you know, for people at agencies and even in-house marketers, when you’re looking at your marketing team, we are all savvy enough to know we should be data driven. And that’s really important. And you’re looking towards those analysts and those doing reporting to help you better understand what happened, who your customer is. But as Lith mentioned, the role of the engineer who’s working in AI and ML is to solve problems and automate decisions. And that is outside of what we as marketers might think where automation happens, which is email platforms, marketing automation platforms. But Lilith, can you share a couple of examples where you’ve automated decision-making and it’s outside of, you know, a marketing automation platform?
Lilith Bat-Leah: Yeah, I’ll focus on, on a couple of examples since I left legal tech, right? Because I, I could be about obviously, but yeah, so one example is a is automating work on a deliverable that we offer as, as a standard part of engagements, right? So, with a lot of our market research or voice of customer work, we would take transcripts of, of interviews and analyze and classify every excerpt, every quote that, that someone said, right? And, and, and really have this thorough analysis of, of every little thing that, that an interviewee or stakeholder would say. This was a really painstaking, tedious process, right? We would record the calls and then use the transcript and copy and paste from the transcript into a spreadsheet. And, and this would take a long time. And in addition to classifying each excerpt, we were also cleaning up the, the text. And that was a pretty tedious manual process. We were able to automate a lot of that, right? First, the, all the copying and pasting from a Word doc to an Excel doc. And then we use the open AI API to, to automate the transcription cleanup and, and just streamline the whole process and still have this great in-depth analysis of everything that was said without nearly as much manual labor.
Tessa Burg: I like that example because it’s a great way to show that the power of ML, automating processes, tedious processes, really results in a higher quality deliverable. Nobody in that process was eliminated from their job.
Lilith Bat-Leah: Oh yeah. It’s very much a human in the loop system, right? Because it’s not perfect. One of the things with machine learning is I I, I never really expect it to be perfect because it is statistics. So you’re always going to have the outliers, you’re always going to have the exceptions. So I do think that a lot of machine learning, the most value you can get out of it is with a human in the loop system, because machines make very different mistakes from the kinds of mistakes that humans make and vice versa, right? So you, you get the most out of it when you have software catching the human mistakes and humans catching the, the machine learning mistakes.
Tessa Burg: I agree. And I think all marketers can relate to performing that type of customer research ahead of doing a campaign. And I should also mention Lilith that you are on our AI Council and the head of the innovations team. And when we look at, you know, that solving that simple problem, the problem of we spend too much time trying to take out these ums and likes, it’d be so much better if we could expedite being able to classify and categorize certain themes together. What are you most excited about as we look at how solving that one problem could also help us solve many more and different kinds of problems for marketers? And how do you approach finding which problem to solve first?
Lilith Bat-Leah: Yeah, that’s a great question. I mean, I, I do think that a lot of the best ideas for innovation come from the people who are closest to the problem, who are closest to, to doing that work. And if they think of, oh, wouldn’t it be great if just, you know, this could happen automatically and then I could do this, or this really tedious part of things was automated and I could spend more time and energy on, on the strategic or creative piece of things. I think a lot of the best ideas just come from the, from the front lines and from being really close to the problem. So engaging everyone, facilitating innovation across the entire organization is a, is a really key piece of that. And, and encouraging people to learn more about how machine learning works under the hood so that they have a better idea of what’s possible and what to expect from, from the technology. I think all of that is really key to, to fostering innovation across the entire organization and industry.
Tessa Burg: Yeah, and I think that discipline of bringing the discipline of data science closer to where the problems are and closer to the people who are on the front lines is relatively new. Like back in the day I was in a data team way long ago in Dayton, Ohio. We sat in a completely separate building from the salespeople, from marketing, from even customer service, yet we were always asked to solve problems out of context. So, it’s really exciting from my experience to see that, you know, you are not only a part of that problem-solving team, but empowered to ask the right questions to better understand the context as to, you know, what do we need to know? What are the right data features to help solve this problem? And I think that the appreciation has always been lower on, you know, how important it’s to select the right kind of data and the right kinds of features to tune a model. To create a model first off, but then to tune it.
Lilith Bat-Leah: Yeah. And the, the value of domain expertise really can’t be overstated. because it is all about knowing the right questions to ask, right? It’s all about asking the, the questions that are going to get you there. And a lot of times if you, if you’re missing that domain expertise, you just don’t know what to ask. You don’t know where you are going to run into the problems with the data or, or what to question or what to doubt or all of those things. So, so I agree that domain expertise is so critical.
Tessa Burg: And speaking of domain expertise, you have another role outside of Mod Op that brings a lot of value to the field in general. Tell us a little bit about your participation in research and the role you play with your, with the nonprofit organization.
Lilith Bat-Leah: Sure. So, so going back to the, the legal tech background in my first R&D role, I hit a point where I realized that so much of what was stopping us in, in terms of, of progress with anything that we were doing on in terms of algorithm or model architecture were data quality issues, right? So, there were examples of things that were just labeled the complete opposite by different attorneys. There was so much inner annotator or disagreement that it made it really, really hard to model what we, what we cared about modeling. So that issue led me to a deep interest in what’s called data-centric AI, where you’re really focused on systematically engineering the data and correcting data issues over and above concerns around model architecture or, or the learning algorithm. So that interest in, in data-centric AI led me to this working group called Data Perf that’s part of ML Commons. And that that group is focused on benchmarking data-centric AI, so benchmarking the best approaches to data selection to what we call data debugging. So, cleaning the noisy labels to data valuation, which dataset is going to allow you to build the, the best model, all of those sorts of problems. And yeah, and I, I’m really, really lucky I get to work with researchers from Harvard, Stanford, Google, Meta, all of these, these people with deep, deep expertise in, in this area and, and push things forward by asking new questions and, and researching different methodologies.
Tessa Burg: And I think that’s critically important for marketers. So, I, maybe not everyone completely followed that answer. We break it down, it gets back to that quality that just because you are capturing data and just because you have a problem to solve does not mean that you might have the right data or it’s well organized, or that it’s in a place to solve said problem and will if you’re investing time outside of work, really helping put standards around what is high-quality data and how do we advance the field in a way that provides greater value to using machine learning AI. I know that you recently participated in a research paper. What kind of sparked the, the need to do that, that paper, and what were some of the main takeaways that you think would be valuable?
Lilith Bat-Leah: Yeah, and so that that paper, it is really exciting. It was accepted at the, the nabs benchmarks and, and dataset track. So, so that is really exciting. It is just kind of a summary of, of all the work that we’ve done as part of Data Perf and it’s looking at a handful of benchmarks that we launched with. And another thing about Data Perf, it’s kind of like, it’s kind of like Google, but for data-centric AI. So rather than iterating on the model, you’re iterating on the data set in in some capacity. So yeah, so it’s a, in, in a lot of the huge advances in machine learning have come from, from benchmarks, from benchmarking. So we thought we really need to start benchmarking data-centric AI in order to help push data-centric AI forward. So that’s where, where that came from. And it’s still evolving. We’re doing some really exciting work with Common Crawl, which is a foundation that provides a lot of the data that is used to train large language models. So right now, we’re working on, on some data quality benchmarking of that data set and, and working with their team to, to come up with challenges that are, that are going to involve training LLMs.
Tessa Burg: You said something before we got on the call that about 80% of machine learning projects fail. And I think a lot of the things we’ve covered today would help, especially marketers increase the success rate. And it is about bringing the domain expertise together with how you’re collecting the data, where you’re collecting it, and then the problem you’re trying to solve and making sure that you’re scoping that problem to one, be efficient and not take on too much, but be specific. What are some other things that you think would help increase the success of ML and companies?
Lilith Bat-Leah: Great question. So, it goes back to asking the right questions and finding the right problems to solve, right? So sometimes something that someone thinks is, is getting at the right problem might actually be skirting around it, right? So it’s, it’s finding the right root causes to address. I know I’m being vague, I wish I could give better, better examples. Sometimes it’s also just thinking through how can this process as a whole change rather than just how can we automate what humans are doing, right? How can we change the way that humans work and empower them with machine learning rather than expecting machine learning to replace them completely or, or trying to do things with the exact same workflow but just replacing the human part with ML, right? So it’s rethinking the whole process, rethinking just how do we get where we’re trying to go without assuming that it’s going to look exactly like it used to, but with one component replaced.
Tessa Burg: Yeah, no, I completely agree. And I think that a lot of marketers are actually struggling with that last piece, that the whole process might have to change. And recognizing that that has nothing to do with your ability. It’s not a reflection of your ability, it’s not a reflection of your domain expertise, it’s a reflection of wanting or needing to get the most out of the investment you’re going to make in an AI ML solution. And that’s across the board, whether you are using an app or building an innovation inside your own company, there has to be this acceptance that how I work today is going to change. And it’s because there is always more than one way to solve a problem. So you may have identified the core problem correctly, but we might be able to get to the answer and best utilize our expertise people and machines by working differently. And I’ve seen that be a blocker. People get very excited about solving the problem. Another one of the awesome solutions that you’ve made that is now available to clients of ours is identifying who’s likely to it’s right. But there also has to be that recognition that there are things we do as a business that, or things we don’t do as a business that contribute to that attrition. So, if we get the answer, we have to accept that we might start operating differently or how we prevent that person from leaving us might be different than what we used to do in the past. So, I think you’re absolutely right that sometimes it’s accepting, you know, that there might, maybe the problem isn’t what you think it is. And then when we do find the problem, and even if it is what you think it is, we’re all going to have to operate a little bit differently. So, when you were doing this research paper back to that, were there any ah-has, because you’ve been so deep in data, did you go in thinking one thing and some unexpected light bulb moments happened?
Lilith Bat-Leah: Yeah, great question. I think we’re still getting there, right? I think we’re still defining what data-centric AI looks like in practice, what the, the approaches are, how we can translate them across different domains and disciplines. But it is really exciting to be part of that nascent area of research. But yeah, hopefully I’ll have more for you there in the near future.
Tessa Burg: All right. So I, if we were to translate this episode into a little blueprint for marketers who are interested in either one using AI and ML applications in their business or to building their own innovation, what, it’s a very similar path and I think some of the key takeaways are one document and really understand the problem you’re trying to solve, but make sure you include a data scientist or some someone from data services with you to help ask different kinds of questions that may just get you to look at your own domain expertise in a different way. And the second thing is, I would say make sure you have some layer of change management involved. Because even if you’re just licensing an app, the way that that app gets used may not be the same way you use the free version because we all interact with different levels of secure data, different other applications that access data in different ways. And the last is, but don’t let this deter you from starting, you know, like think it, all of our previous episodes have focused on that AI and ML will change the way that we work. And so, partnering with a great data scientists can help you see where that change is happening more efficiently and increase your success and execution. Lilith, you have been now in the marketing space for how many, like two years, three years or I guess dPrism was very much more in digital transformation but still helped create the data, data architecture and tech stack that marketing would make use of. Is there anything else you’ve observed that you think marketers should take into consideration as, you know, this really is a new frontier, but one that will continue to accelerate?
Lilith Bat-Leah: Yeah, maybe that old adage of ‘don’t let perfect be the enemy of good,’ right? So when considering solutions it’s all about, well is it better than the baseline? Is it better? Does it achieve better results than what we’re doing now? And yeah, it may not be perfect, but neither are humans or, or existing processes. So, giving technology a chance and, and yeah, and just trying to, to push things forward even by, by some small margin I think is really critical right now.
Tessa Burg: Yeah, I love that. And I would say we’re going to end there, but there was something that happened yesterday. Lilith and I were on a meeting with another senior-level person in the data field and it struck me that there were, you know, three women talking about data science and compliance and technology. And for myself, that was the first time that that hadn’t happened in my career. And Lilith said something earlier that really resonated with me and made me realize how much I love data and it’s the love of statistics and or you know, Python. It’s the love of wanting to build something. And I think that if you’re sitting in a seat right now and you are an analyst at your current marketing job or you have just started learning Python because you heard that, that it was a way to get to know AI and ML a little bit better, that there is an opportunity to explore that. And if you are a female and maybe that didn’t feel like it was available to you, but it very much is because it’s more about, you know, what drives your passion and curiosity than it is if you’re good at math or science. Which I know a lot of us were told girls aren’t as good at math and science. I think statistics is, for me feels wildly different than like straight up math. So yeah, everyone, well do you have anything to add to that?
Lilith Bat-Leah: Yeah, no, I, I completely agree. I think, I think this stuff is more accessible than it ever has been and there are all these emerging roles. Yeah, so there’s, there’s the role of AI engineer where you’re really just working with the APIs for these foundation models and right in that actually doesn’t require as much math or statistics. But if you’re building models from scratch like that, that churn prediction model that we discussed a little bit earlier, that’s where it is really important to, to have a super solid foundation of, of math and statistics in order to, to really understand the technology that you’re using.
Tessa Burg: Yeah, and I’m glad you pointed out that there are a lot of roles that really are in and around that heavy math and statistics role and I think are the key or a big part of AI and ML being successful because it makes the models or the value of the models are creating more available to the people who are actually using them and experiencing the challenges and issues. So if you are a person who is, you know, you’re not going to get deep into math statistics but you understand it, you like it, you love building your domain expertise can actually be the thing that differentiates you in one of those, I don’t want to say in between roles, but one of those bridge roles that helps get the AI and ML to where it’s truly adding value to the business and to the users.
Lilith Bat-Leah: 100%. Yeah.
Tessa Burg: Well thank you so much for being our guest today. It has been a joy getting to work with you on the AI Council and be following your journey. If people would like to get in touch with you and learn more, where can they, where can they find you?
Lilith Bat-Leah: Thank you so much for having me, Tessa. Probably LinkedIn is the best place. So yeah, feel free to reach out
Tessa Burg: And LinkedIn if they search Bat-Leah, that’s hyphenated. BAT dash LEAH, Lilith Bat-Leah. And then you can also find out more about what Mod Op is doing and how we’re leveraging our data services team to create innovation to help our clients expedite their use of AI and ML. Visit us at modop.com and you can listen to more Leader Generation episodes. That is all for this week. Until next time, thanks for listening.
Lilith Bat-Leah
Vice President of Data Services at Mod Op
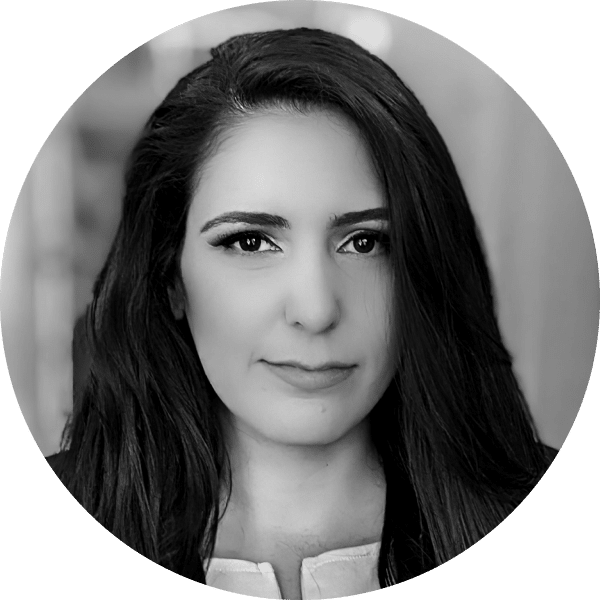
Lilith is responsible for strategic consulting on use cases for data analytics, data science and machine learning. She has more than 11 years of experience managing, delivering and consulting on the identification, preservation, collection, processing, review, analysis and production of digital data. Lilith also has experience in research and development of machine learning software. She specializes in the application of statistics, analytics, machine learning and data science to natural language and other unstructured data. She is passionate about making impossible things possible and is driven by curiosity.